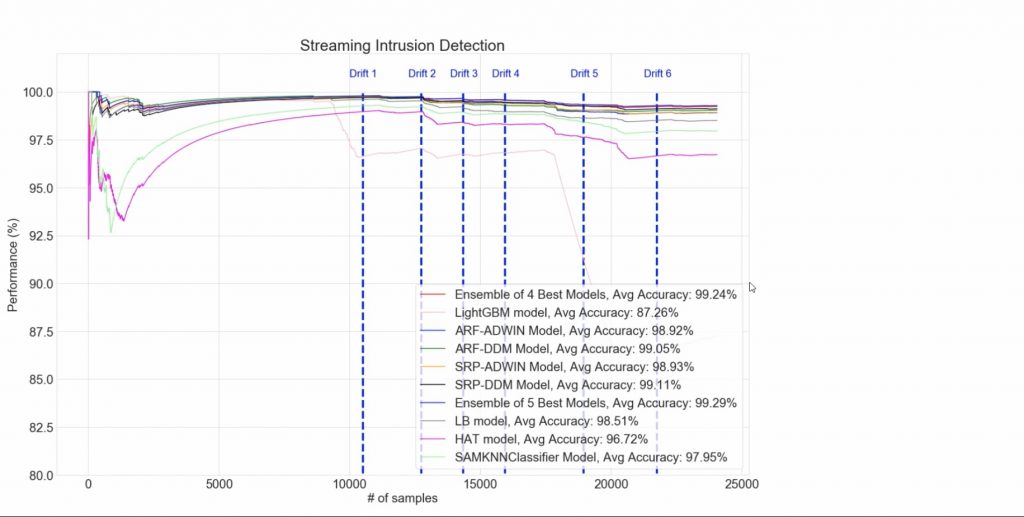
Advanced network Intrusion Detection Systems (IDS) utilise the power of machine learning (ML) and big data technologies. Various machine learning methods and tools have been developed with the purpose to identify anomalous behaviour, detect and classify intrusions. Every method has advantages and disadvantages and its performance may vary widely depending on the type of cyberattack.
IDS Combo is developed by a team at the Institute of Information and Communication Technologies of the Bulgarian Academy of Sciences, with Petya Ivanova as lead developer. The tool’s name originates from IDS Combined Methods Toolbox. It builds on the advances in machine learning and available technologies and capacity to collect, store, and process large amounts of historical and current data in near real-time. As the name suggests, it combines the power of the most promising machine learning methods for data pre-processing, feature engineering, identification of anomalous behaviour, and classification of attacks. The hypothesis underlying IDS Combo is that using an ensemble of carefully selected methods will provide for increased accuracy and performance in identifying and classifying intrusion attempts.
Within the timeframe of the ECHO project, the research team proved this hypothesis. For that purpose, it created a comprehensive framework based on developments in the field of Artificial Intelligence and big data analytics. Proven and emerging ML methods were implemented in a coherent process of design and development of an optimized intrusion detection system. This process spans the whole chain of important design considerations – the ‘representation’ problem (design of the feature space), design, training and testing of relevant models; the output space (where the outputs of an ensemble of different methods are combined); identification of statistically significant changes of the threat landscape (called also ‘concept drift’) and adaptation of the whole chain of models to identified drifts; and enhancing the explanation of the tool outputs to a human expert.
The figure below depicts the core of IDS Combo.
For the proof of concept, the research team identified, optimised and tested dozens of relevant ML methods, utilising several open datasets, widely used in benchmarking studies of network intrusion detection methods and models. The University of Brunswick’s CSE-CIC-IDS2017 dataset was used for the full testing of the tool and validation of the hypothesis.
The readiness level of IDS Combo reached during the ECHO project is TRL3+. Thus, we proved that the combined use selected ML methods and models developed for anomaly detection and classification allows to go beyond the current state-of-the-art by:
- advanced feature engineering providing for processing bigger amounts of data in real time without degrading the intrusion detection performance;
- identification of novel types of attacks and changing attack distribution and adaptation of the tool to such concept drifts;
- enhancing the explainability of the tool outputs to human experts (e.g. system administrators and data scientists).
In mid-term, IDS Combo will evolve into an easy to deploy, self-adapting tool for network intrusion detection, using stored and incoming data and alerts from numerous relevant sources and state-of-the-art machine learning and other artificial intelligence methods to detect and classify quickly novel and emerging cyber threats. The tool will possibly be integrated with internal threats’, intrusion prevention, and other tools in a comprehensive cybersecurity system, informing in real-time its users on status and required actions.
If you wish to know more about IDS COMBO, watch the demonstration video on our YouTube channel: